Generative AI Funding Hits $25.2 Billion in 2023, Report Reveals
Stanford’s AI Index report reveals a nearly eightfold increase in generative AI funding
.jpg?width=850&auto=webp&quality=95&format=jpg&disable=upscale)
At a Glance
- Stanford's 2024 AI Index highlights a shift in AI investment patterns, with generative AI attracting $25.2 billion.
- Foundation model training costs also rose, with companies including OpenAI, Google spending millions on new technologies.
Funding in the generative AI space surged dramatically in 2023 as major players including OpenAI and Anthropic recorded substantial increases in capital, according to a new report from Stanford University.
Stanford’s 2024 AI Index revealed a nearly eightfold increase in funding for generative AI firms, soaring to $25.2 billion in 2023.
The report found that generative AI accounted for more than one-quarter of all AI-related private investment in 2023.
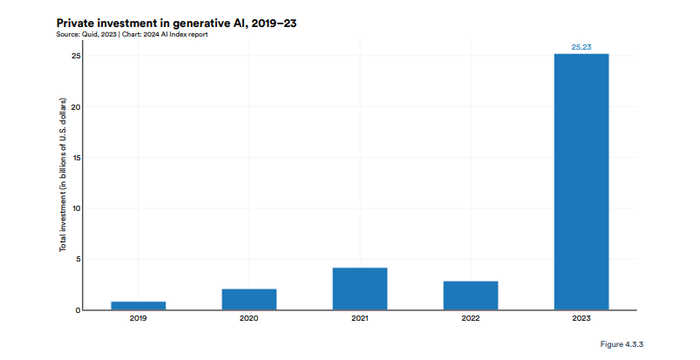
Credit: Stanford HAI
Last year’s major investments included Microsoft’s $10 billion OpenAI deal, Cohere’s $270 million raise in June 2023 and Mistral’s $415 million funding round in December among a host of others.
Stanford’s report noted, however, that corporate spending on AI dropped in 2023, decreasing by 20% to $189.2 billion.
The report attributed the dip to a reduction in mergers and acquisitions which fell 31.2% from the previous year. Despite the drop, nearly 80% of earnings calls for Fortune 500 firms mentioned AI.
Investments were dominated by firms from the U.S., with $67.2 billion invested, almost nine times greater than the amount invested by the second-highest spender, China with $7.8 billion.
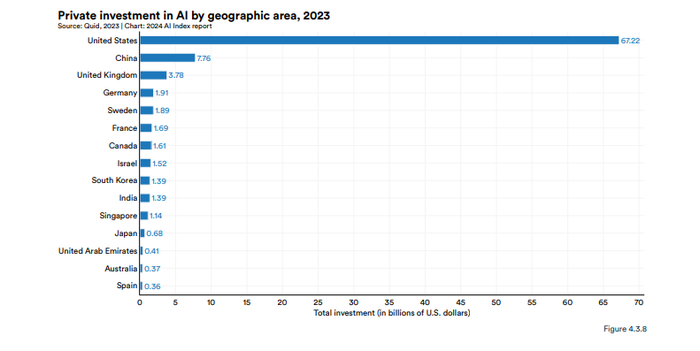
Credit: Stanford HAI
The report found that private investment in AI dropped in China and the EU in 2023 compared to 2022, while U.S. spending rose by 22.1%.
AI spending in the U.S. is also having an impact on salaries. Stanford’s report referenced figures from a Stack Overflow survey which found that salaries for AI roles are significantly higher in the U.S. than in other countries.
For example, the average salary for a U.S.-based hardware engineer in 2023 was $140,000 while globally it was $86,000. The global average for a cloud infrastructure engineer is $105,000, while such a role commands $185,000 in the U.S.
The areas that attracted the most investment in 2023 were AI infrastructure, research and governance with $18.3 billion. Stanford’s report stated that this spending reflected big players including OpenAI and Anthropic building large-scale applications like GPT-4 Turbo and Claude 3.
The second highest spending sector was natural language processing and customer support $8.1 billion, as businesses look to adopt solutions to augment workflows through use cases like automating contact centers.
The U.S. was the largest spender across all the various AI technology categories bar facial recognition where China came out on top, spending $130 million compared to $90 million in the U.S.
In terms of semiconductor spending, Stanford found China ($630 million) was not far behind the U.S. ($790 million). Global governments have been increasing semiconductor spending to shore up supply chains following the 2020 global hardware chip shortage.
Foundation Models Grow in Cost
With companies like OpenAI raising millions of dollars in funding, Stanford’s report notes that those same firms are raking up big bills from training new models.
Model training costs rose in 2023, with Stanford’s researchers suggesting investments in large-scale foundation systems were a leading factor behind the rise.
The AI Index reported that the training costs for advanced AI models have risen significantly. For example, OpenAI spent an estimated $78 million to train its GPT-4 model, while Google's flagship Gemini model required an estimated $191 million.
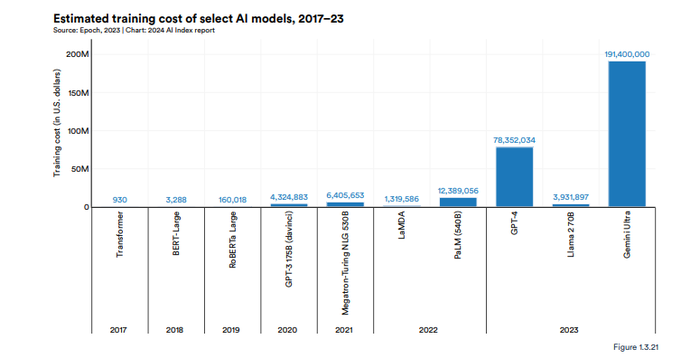
Credit: Stanford HAI
In contrast, earlier models were much less expensive: the original Transformer model, released in 2017, cost around $900 to train and Facebook’s RoBERTa Large system from 2019 cost approximately $160,000.
Model developers seldom publish figures on model training spending. Stanford collaborated with Epoch AI to come up with estimates on training costs. The estimates were based on information from related technical documents and press releases and encompassed an analysis of training duration as well as the type, quality and training hardware’s utilization rate.
In addition to costing millions to train, AI models trained in the past year used more training compute. The report notes that Google’s 2017 Transformer model demanded around 7,400 petaFLOPs to train. Seven years later, Gemini Ultra required 50 billion petaFLOPs.
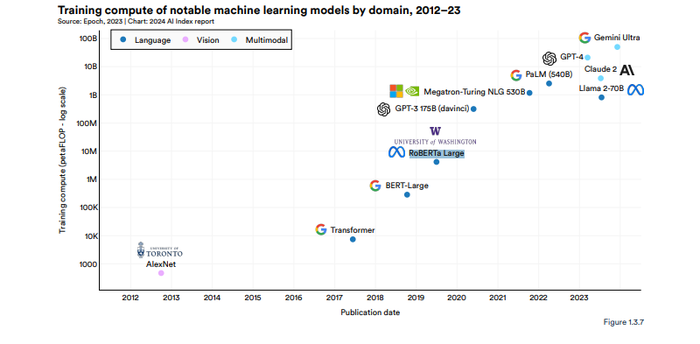
Credit: Stanford HAI
Stanford warns that these power-intensive systems like Gemini Ultra are becoming increasingly inaccessible to academia due to their sheer costs involved to run them.
“This shift toward increased industrial dominance in leading AI models was first highlighted in last year’s AI Index report. Although this year the gap has slightly narrowed, the trend largely persists,” according to the report.
Google is the company releasing the model foundation models, publishing 40 since 2019. OpenAI stands in second with 20. The top non-Western outlet releasing AI models was China’s Tsinghua University with seven.
The majority of large-scale AI systems published in 2023 stemmed from the U.S., with 109. Chinese institutions were second, but only managed 20. The U.S. has been the top producers of AI models since 2019, Stanford’s report notes.
One growing trend highlighted in the report was the growing number of multimodal AI models or systems that can process images or videos as well as text.
"This year, we see more models able to perform across domains," said Vanessa Parli, Stanford HAI director of research programs. "Models can take in text and generate audio or take in an image and generate a description. An edge of the AI research I find most exciting is combining these large language models with robotics or autonomous agents, marking a significant step in robots working more effectively in the real world."
Read more about:
ChatGPT / Generative AIAbout the Author(s)
You May Also Like